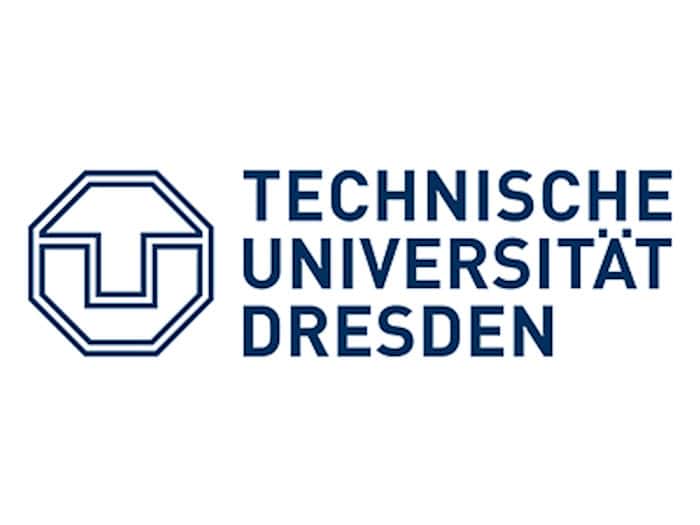
Model development using common multilingual benchmarks
While most available benchmarks for evaluating language models are predominantly available for the English language, the OpenGPT-X consortium has set itself the goal of comprehensively expanding language accessibility for multilingualism and thus paving the way for fairer and more effective language technology. To reduce language barriers in the digital domain, the scientists carried out extensive multilingual training runs and then tested the developed AI models with regard to tasks such as logical reasoning, commonsense understanding, multitasking learning, truth content and translation skills.
When developing LLMs, it is important that training and evaluation go hand in hand. To enable comparability across multiple languages, some of the most common benchmarks such as ARC, HellaSwag, TruthfulQA, GSM8K and MMLU were machine translated into 21 of the 24 supported European languages using DeepL. In addition, two further multilingual benchmarks were added to the leaderboard, which were already available for the languages considered in the project.
The plan is to use the leaderboard to automate the evaluation of models from the AI platform Hugging Face Hub in order to enable the traceability and comparability of the results. TU Dresden will provide the necessary infrastructure for this and carry out the evaluation jobs on the HPC cluster. Following the current release of the European LLM Leaderboard, the OpenGPT-X models will be published this summer and will also be visible there. This is because one of the core goals of OpenGPT-X is to make the benefits of these AI language models available to a wider audience in Europe and beyond and to support a large number of European languages. This progress is particularly important for languages that are traditionally underrepresented in the field of natural language processing.
TU Dresden with bundled big data, AI and HPC expertise in the project
With the expertise of the two competence centers ScaDS.AI (Scalable Data Analytics and Artificial Intelligence) and ZIH (Information Services and High Performance Computing) at TU Dresden, OpenGPT-X has a cooperation partner that pools expertise in training and evaluating large language models on supercomputing clusters. The joint efforts will focus on several critical tasks, including developing scalable evaluation pipelines, integrating various benchmarks, and performing comprehensive evaluations on supercomputing clusters. The team also focuses on improving model performance, scalability and efficiency, continuously monitoring the impact of pre-training and fine-tuning it, and leveraging innovative high-performance computing resources.
Overview of the benchmarks translated in the project
ARC (https://huggingface.co/datasets/ai2_arc) and GSM8K (https://huggingface.co/datasets/openai/gsm8k) focus on general education and math.
HellaSwag (https://huggingface.co/datasets/Rowan/hellaswag) and TruthfulQA (https://huggingface.co/datasets/truthfulqa/truthful_qa) test the ability of models to provide plausible continuations and truthful answers.
MMLU (https://huggingface.co/datasets/cais/mmlu) provides a wide range of tasks to assess the ability of models to perform in a variety of domains and tasks.
While FLORES-200 (https://huggingface.co/datasets/facebook/flores) focuses on assessing machine translation skills, Belebele (https://huggingface.co/datasets/facebook/belebele) focuses on understanding and answering questions in multiple languages.
Funding, project management and contacts
The OpenGPT-X project has been funded by the BMWK since 2022 and is largely coordinated by the Fraunhofer Institute for Intelligent Analysis and Information Systems (IAIS).
Contacts
IAIS – Dr. Nico Flores-Herr, Dr. Michael Fromm
TU Dresden, ScaDS.AI: Dr. René Jäkel, Klaudia-Doris Thellmann
Publications
- Ali, Mehdi, Fromm, Michael, Thellmann, Klaudia, Rutmann, Richard, LĂĽbbering, Max, Leveling, Johannes, Klug, Katrin, Ebert, Jan, Doll, Niclas, Buschhoff, Jasper, Jain, Charvi, Weber, Alexander, Jurkschat, Lena, Abdelwahab, Hammam, John, Chelsea, Ortiz Suarez, Pedro, Ostendorff, Malte, Weinbach, Samuel, Sifa, Rafet, Kesselheim, Stefan, & Flores-Herr, Nicolas. (2024). Tokenizer Choice For LLM Training: Negligible or Crucial? In K. Duh, H. Gomez, & S. Bethard (Eds.), Findings of the Association for Computational Linguistics: NAACL 2024 (pp. 3907-3924). Mexico City, Mexico: Association for Computational Linguistics. Retrieved from https://aclanthology.org/2024.findings-naacl.247
- Weber, Alexander Arno, Thellmann, Klaudia, Ebert, Jan, Flores-Herr, Nicolas, Lehmann, Jens, Fromm, Michael, & Ali, Mehdi. (2024). Investigating Multilingual Instruction-Tuning: Do Polyglot Models Demand for Multilingual Instructions? arXiv. Retrieved from https://arxiv.org/abs/2402.13703
– – – – –
Further links
👉 www.tu-dresden.de
Photo: TU Dresden