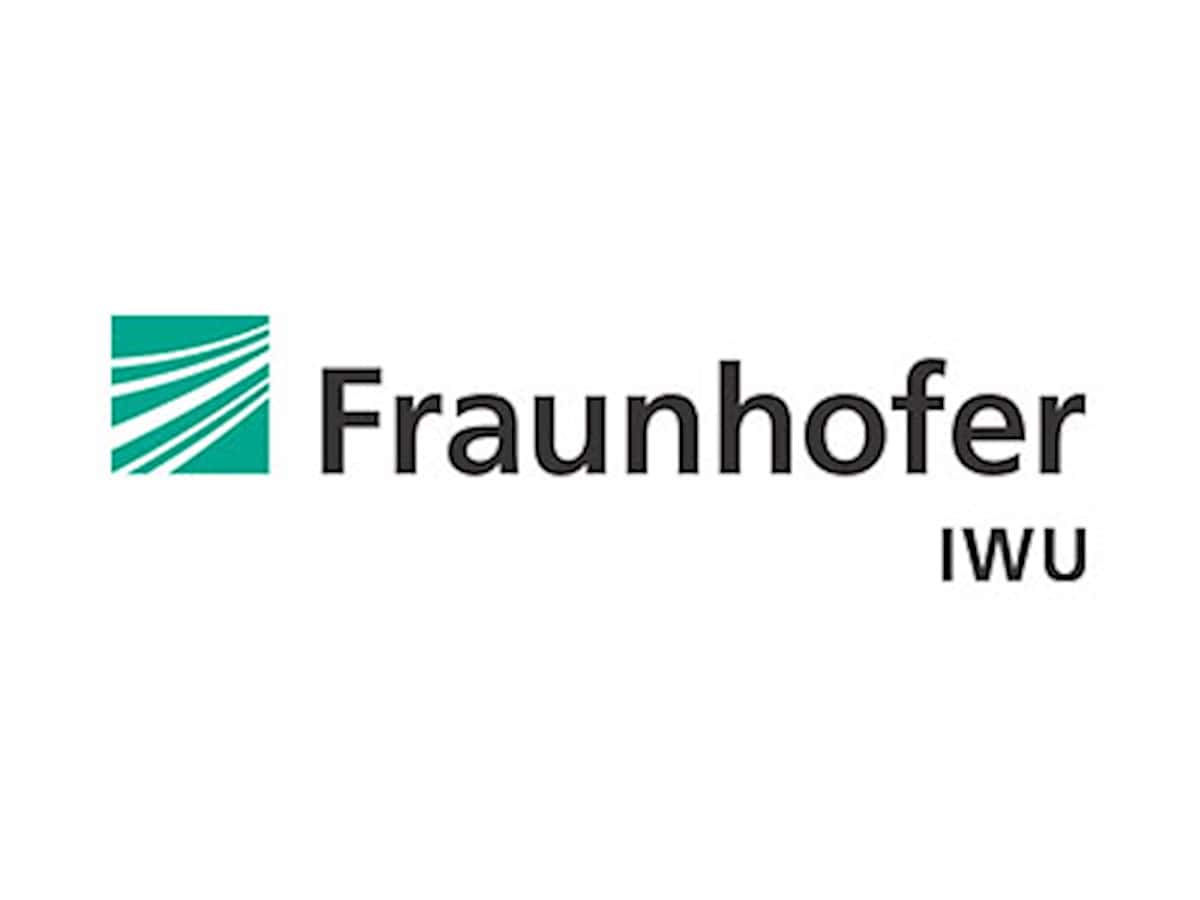
Large language models (LLMs) often deliver good to very good results in chatbots for everyday queries. The answers are usually more than sufficient for initial orientation. However, you should also be aware of their limitations: Training data sets may be incomplete or outdated, some information may be fuzzy or even incorrect. It is therefore advisable to check the information received. When it comes to legal issues, you should not ‘blindly’ rely on a chatbot. So what should you do if the safety of people could depend on the information provided? Should you study the relevant documents in detail again?
RAG ensures reliable statements on the EU Machinery Regulation
This doesn’t have to be the case – if additional guard rails are added to the language model using RAG. It then primarily screens relevant texts or text passages. The language model is not retrained, but selectively expanded. As an example, Fraunhofer IWU is now incorporating the EU Machinery Regulation (2023/1230) into an LLM.
Operation should also be possible on standard PCs
The choice for a suitable model fell on LLaMA (Large Language Model Meta AI), which is large or powerful enough and yet does not overtax the computing power and graphics card of a high-quality standard PC. The application can therefore be run on a local computer. In this case, companies retain complete control over their data. For less sensitive data, operation in the cloud is also possible.
How RAG works: several steps to fact-based answers
First, the data to be imported into LLM must be reduced to pure text (cleaning). As soon as this has been segmented into smaller sections (chunks; retrievable building blocks), the next step is to set up a retrieval system that can search the chunks efficiently. The chunks are organized according to relevant passages and stored in a vector database, i.e. converted into mathematical vectors that represent their meaning. The prompts are also converted into vectors. This enables the model to search for the words contained in the query and at the same time actually ‘understand’ the prompt (semantic search). The model can now shorten the chunks that match a user query, restructure them, filter out the most important information and combine them into a comprehensible context. If there is a specific search query, selected chunks are available on the basis of which the model can provide fact-based answers. The model uses the additional context of the chunks and does not need to be retrained.
The right structure makes all the difference
Machine tools are one of IWU’s core competencies. The ‘Machine Learning in Production’ team knows which filters and pre-structuring are important in order to reach the decisive passages of the machine ordinance. In future, an important focus will also be on other data sources such as tables or images, which must be integrated in such a way that they are easy to find.
Smart AI tool for legal texts, operating instructions, procedural instructions…
The Machinery Regulation is intended to ensure uniform standards within the EU. For example, it defines when a new conformity assessment is required for changes to machinery and systems. As an example of a demanding legal text, it is the starting point for the demonstration applications at the IWU. Such texts need no longer frighten small or medium-sized companies that do not have large teams of experts at their disposal: The team of experts from Chemnitz offers its methodological expertise to build tailor-made applications with interested companies. This could include operating instructions, the preparation of quotations or the automation of programming work in the production context as well as ‘tiresome’ reporting obligations. Searching through internal company documents, regulations, company agreements or operational data can also be supported by AI in the future.
– – – – – –
Further links
👉 www.iwu.fraunhofer.de
Photo: Image generated with AI (Adobe Firefly)