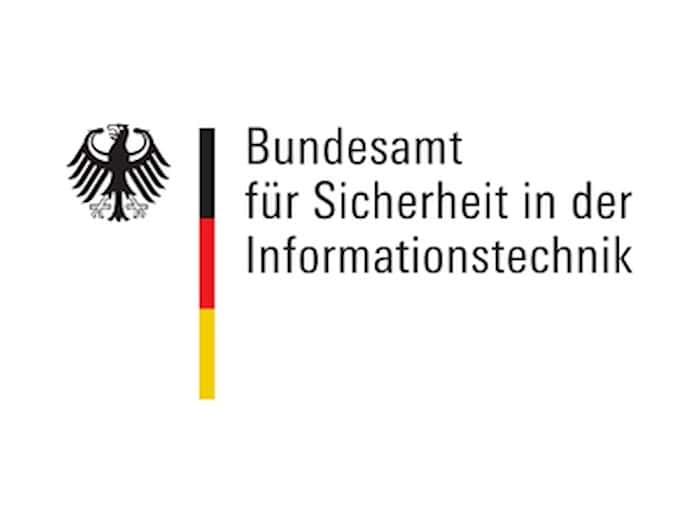
Transparency for black box models through post-hoc methods
XAI aims to make the decision-making processes of AI systems comprehensible. Many AI models, especially those based on deep learning, act as a “black box” whose internal processes are difficult to understand. The BSI white paper focuses on post-hoc methods that provide subsequent explanations for these black box models and analyze the influence of individual features on decisions.
Challenges and opportunities of XAI
Although XAI offers opportunities for gaining knowledge and optimizing models, there are also challenges, such as the problem of disagreement and the susceptibility of explanations to manipulation. The explainability of AI is crucial for trust in these technologies and helps developers and users to better understand how they work. Nevertheless, developing standardized methods to consistently ensure explainability remains a key challenge.
– – – – – –
Further links
👉 www.bsi.bund.de
👉 Explainability of AI in an Adversarial Context (PDF)
👉 Explainable Artificial Intelligence in an Adversarial Context (PDF)
Photo: pixabay